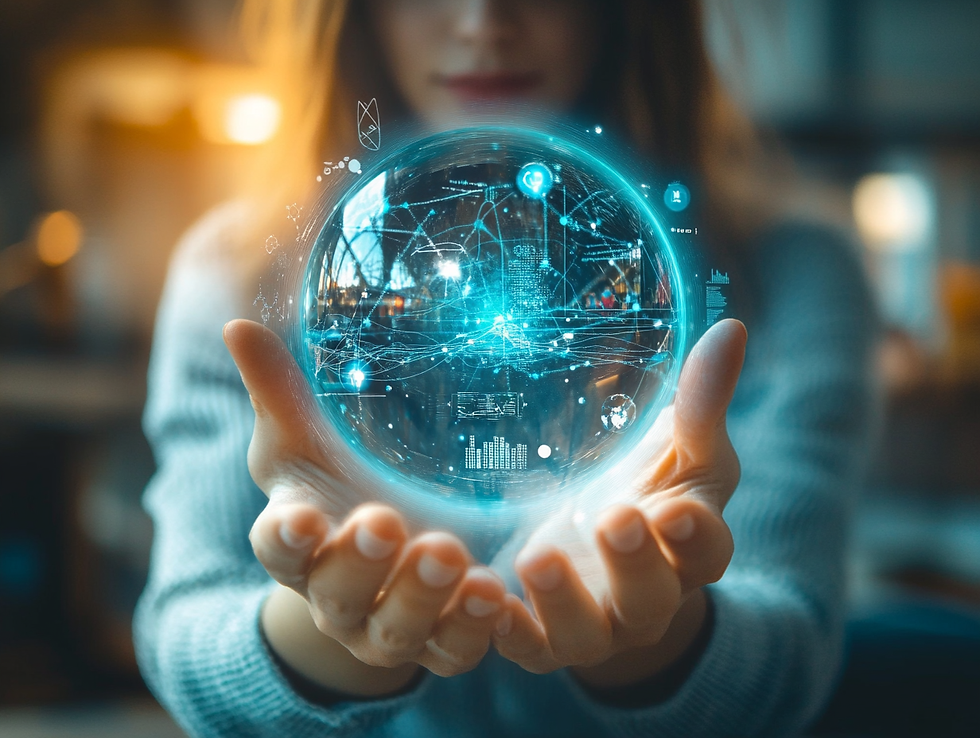
I'm having an eighties flashback today. Using a storytelling device made popular in my youth, I will guide you through the perhaps not-so-fascinating world of predictive analytics in mortgage banking using the fictional story of Regional Bank. Regional Bank is a made-up institution designed to showcase the myriad ways predictive analytics can be implemented free from the constraints of reality.
Through this narrative, we’ll explore how AI-driven insights can transform risk assessment, loan origination, customer retention, fraud detection, and more, all while maintaining ethical standards and ensuring regulatory compliance. This is an object lesson in what’s possible when you harness the full potential of predictive analytics in the mortgage industry. So, let’s dive into the story of Regional Bank and see how they’re leading the charge with innovative, data-driven strategies.
Geaux Regional Bank
Regional Bank turned to predictive analytics to stay ahead of the competition and enhance its decision-making capabilities in mortgage banking. They needed a sophisticated approach to uncover patterns in vast datasets. Partnering with a fintech firm, they developed an AI-driven predictive analytics model. This model analyzed diverse data sources, including financial histories, employment records, income stability, spending patterns, weather trends, and even social media activity, to provide a comprehensive risk profile for each borrower.
Predictive analytics is reshaping mortgage banking, and Regional Bank’s journey provides a detailed view of its practical application. By integrating AI-driven models into their processes, Regional Bank has enhanced decision-making, streamlined operations, and maintained a competitive edge. This story serves as an object lesson, illustrating how the ethical and strategic implementation of predictive analytics can drive success in the mortgage industry.
Advanced Risk Assessment
Consider John, a freelancer with variable income but a consistent history of timely payments. Traditional models might classify him as high risk due to income instability. However, the AI model at Regional Bank identified patterns in John’s financial behavior that indicated reliability, allowing the bank to offer him favorable loan terms. This nuanced risk assessment contributed to a significant reduction in default rates within the first year.
The AI model's capabilities extend beyond simple risk scoring. It involves complex machine learning algorithms, such as gradient boosting machines (GBMs) and neural networks, to analyze non-linear relationships within the data. These algorithms are trained on historical loan performance data, capturing intricate patterns and trends that traditional linear models might miss. Using techniques like feature engineering, the model can derive new predictors from existing data, enhancing its predictive power.
Streamlining Loan Origination
The loan origination process, known for its complexity and delays, benefited significantly. Regional Bank’s AI model evaluated potential borrowers in real-time, enabling instant pre-qualification. For instance, Mary, a first-time homebuyer, could secure pre-qualification during a lunch break, thanks to the AI-driven system. Integrating predictive analytics into the loan origination workflow gave loan officers real-time recommendations on suitable loan products, increasing conversion rates and reducing processing times. Automated checks for document completeness and consistency minimized manual errors and processing delays.
This efficiency gain was achieved through natural language processing (NLP) algorithms, which processed and verified documents quickly, ensuring compliance with regulatory standards. Optical character recognition (OCR) technology was employed to digitize and extract information from paper documents, feeding this data into the predictive models for real-time analysis.
Enhancing Customer Retention and Acquisition
Customer retention and acquisition, critical for growth, saw marked improvements through predictive analytics. By analyzing customer behavior, Regional Bank could predict which customers were likely to leave and why. This allowed the bank to intervene with personalized offers and improved services, maintaining customer loyalty. For instance, when the system detected that Michael, a long-time customer, was comparing competitor rates, Regional Bank swiftly provided a tailored refinancing offer, securing his continued business.
For customer acquisition, the bank utilized clustering algorithms to segment its customer base and identify high-potential leads. Techniques like k-means and hierarchical clustering helped group customers based on similar characteristics, such as financial behavior, demographic data, and engagement levels. This segmentation allowed for more targeted marketing efforts, leading to an increase in new customer acquisition. A churn prediction model also facilitated tailored retention strategies, reducing churn rates.
Fraud Detection and Prevention
Fraud detection, an ever-present concern, was significantly enhanced. The AI-driven real-time analysis allowed Regional Bank to detect anomalies indicative of fraud. For example, when multiple loan applications originated from the same IP address in a short timeframe, the system flagged it for further investigation. Early detection and intervention reduced fraud-related losses significantly.
The fraud detection system relied on unsupervised learning techniques, such as anomaly detection and clustering algorithms, to identify unusual behavior patterns. These models continuously learned from new data, improving their accuracy over time. The integration of supervised learning techniques, such as logistic regression and decision trees, helped to classify transactions as fraudulent or non-fraudulent based on historical fraud data.
Optimizing Loan Pricing
Loan pricing optimization was another area where predictive analytics made a difference. By analyzing market trends, borrower behavior, and competitive pricing, Regional Bank set optimal interest rates that balanced profitability and attractiveness to borrowers. This dynamic pricing model adjusted in real-time to market fluctuations, ensuring that Regional Bank remained competitive while maximizing margins.
The pricing model used advanced regression techniques and time-series analysis to forecast interest rate trends and adjust loan pricing accordingly. This involved integrating external economic indicators, such as inflation, unemployment, and housing market trends, into the predictive model. By continuously monitoring these indicators, the bank could dynamically adjust loan pricing to reflect current market conditions.
Enhancing Portfolio Management
In portfolio management, predictive analytics provided insights into loan performance, identifying trends and potential risks. This enabled Regional Bank to proactively manage its portfolio, adjusting strategies to mitigate risks and capitalize on emerging opportunities. For instance, the bank identified segments with higher default risks and took preemptive measures to reduce exposure.
The portfolio management system used predictive analytics to model the impact of various economic scenarios on loan performance. Techniques like Monte Carlo simulations and stress testing were employed to evaluate the loan portfolio's resilience under different market conditions. This allowed the bank to develop strategies for risk mitigation, such as adjusting loan loss provisions and diversifying the loan portfolio.
Ensuring Regulatory Compliance
Predictive analytics significantly streamlined compliance and regulatory reporting. The tools continuously monitored transactions and activities for signs of non-compliance, generating real-time reports that ensured the bank met all regulatory requirements. This automated compliance reduced the burden on staff and maintained customer trust.
The compliance system used machine learning algorithms to analyze transaction data and identify patterns indicative of regulatory breaches. By integrating with the bank's existing compliance framework, the system could automatically generate reports and alerts, promptly addressing any potential issues. This proactive approach to compliance helped Regional Bank avoid regulatory fines and maintain a strong reputation in the market.
Managing Operational Risks
Predictive analytics also mitigated operational risks, such as system failures or cybersecurity threats. By analyzing patterns and anomalies, the bank could predict potential operational issues and take proactive measures to prevent them. This enhanced the bank’s resilience and ensured smooth, uninterrupted operations.
The operational risk management system used predictive maintenance techniques to monitor the health of IT infrastructure and detect early signs of system failures. Machine learning models analyzed historical system performance data to predict potential issues, allowing the IT team to address them before they escalated. Additionally, cybersecurity threats were mitigated using anomaly detection algorithms that identified unusual network activities and potential breaches in real time.
Ethical Implementation of Predictive Analytics
Implementing predictive analytics requires careful consideration of ethical issues, particularly data bias and fairness. Regional Bank was committed to ensuring its predictive models were free from biases that could lead to discriminatory practices. They implemented rigorous testing and validation processes to identify and mitigate biases in their models. Techniques like fairness-aware machine learning were employed to ensure that predictions were equitable across different demographic groups.
The bank also prioritized transparency in its use of predictive analytics. Customers were informed about how their data was being used, and the bank ensured that all data collection and processing practices complied with privacy regulations. This commitment to ethical practices helped build customer trust and reinforced the bank’s reputation for integrity.
Strategic Implementation
Implementing predictive analytics presented challenges, primarily around data quality and integration. The bank had to clean and consolidate data from various sources to ensure consistency and accuracy. Continuous refinement of models was necessary to enhance accuracy and mitigate biases. Regulatory compliance, particularly regarding personal data use, requires careful adherence to maintain customer trust and avoid penalties.
Regional Bank tackled these challenges head-on. They started by clearly defining their objectives and investing in robust data warehousing solutions to consolidate data from multiple sources. They selected a scalable, cloud-based predictive analytics platform. Collaborating with data scientists, they developed and continuously updated custom models. A dedicated team monitored and maintained these models to ensure ongoing effectiveness and adaptability.
By leveraging predictive analytics, Regional Bank improved its risk management and loan origination processes, customer acquisition and retention, fraud detection, loan pricing, and compliance. The result was a significant boost in profitability and a stronger competitive position in the market.
AI-driven predictive analytics isn’t just a tech buzzword; it’s a powerful tool that can provide a competitive edge in mortgage banking. By leveraging data-driven insights, banks can make more informed decisions, enhance customer experiences, and drive profitability. The real question is, how quickly can you integrate it into your operations? The competitive advantage it offers is too significant to ignore. Embrace the future, harness the power of AI-driven predictive analytics, and watch your mortgage banking operations thrive.
Conclusion to Predictive Analytics in Mortgage Banking
Regional Bank’s journey with predictive analytics demonstrates the transformative potential of AI in the mortgage industry. From advanced risk assessment to streamlined loan origination, enhanced customer retention, and robust fraud detection, predictive analytics offers myriad benefits. The key to success lies in strategic implementation, continuous model refinement, and a commitment to leveraging data-driven insights to drive decision-making. As Regional Bank has shown, the future of mortgage banking is bright for those willing to embrace the power of AI and predictive analytics.
Progressive thinking. The future is here.